"Towards an atlas of variant effects" Article Collection
Published on July 3rd, 2023 in Genome Biology
This collection published on July 3rd, 2023 in Genome Biology, titled "Towards an atlas of variant effects” highlights the power of approaches of Multiplex assays of variant effect (MAVEs) as well as computational tools for understanding gene function, disease variants and biology.
The stage for this collection of articles is set by a short correspondence piece from the Atlas of Variant Effects (AVE) Alliance. In the perspective, we outline our vision and specific approach for creating a comprehensive Atlas, which would characterize the function of every possible single nucleotide change in most genes in the human genome.
Articles in the collection include
Mapping MAVE data for use in human genomics applications.
Genome biology 2025;26;1;179
Experimental data from functional assays have a critical role in interpreting the impact of genetic variants. Assay data must be unambiguously mapped to a reference genome to make it accessible, but it is often reported relative to assay-specific sequences, complicating downstream use and integration of variant data across resources. To make multiplexed assays of variant effect (MAVE) data more broadly available to the research and clinical communities, the Atlas of Variant Effects Alliance mapped MAVE data from the MaveDB community database to human reference sequences, creating an extensive set of machine-readable homology mappings that are incorporated into widely used human genomics applications.
An Atlas of Variant Effects to understand the genome at nucleotide resolution.
Genome biology 2023;24;1;147
Sequencing has revealed hundreds of millions of human genetic variants, and continued efforts will only add to this variant avalanche. Insufficient information exists to interpret the effects of most variants, limiting opportunities for precision medicine and comprehension of genome function. A solution lies in experimental assessment of the functional effect of variants, which can reveal their biological and clinical impact. However, variant effect assays have generally been undertaken reactively for individual variants only after and, in most cases long after, their first observation. Now, multiplexed assays of variant effect can characterise massive numbers of variants simultaneously, yielding variant effect maps that reveal the function of every possible single nucleotide change in a gene or regulatory element. Generating maps for every protein encoding gene and regulatory element in the human genome would create an 'Atlas' of variant effect maps and transform our understanding of genetics and usher in a new era of nucleotide-resolution functional knowledge of the genome. An Atlas would reveal the fundamental biology of the human genome, inform human evolution, empower the development and use of therapeutics and maximize the utility of genomics for diagnosing and treating disease. The Atlas of Variant Effects Alliance is an international collaborative group comprising hundreds of researchers, technologists and clinicians dedicated to realising an Atlas of Variant Effects to help deliver on the promise of genomics.
PUBMED: 37394429 PMC: PMC10316620 DOI: 10.1186/s13059-023-02986-x
Guidelines for releasing a variant effect predictor.
Genome biology 2025;26;1;97
Computational methods for assessing the likely impacts of mutations, known as variant effect predictors (VEPs), are widely used in the assessment and interpretation of human genetic variation, as well as in other applications like protein engineering. Many different VEPs have been released, and there is tremendous variability in their underlying algorithms, outputs, and the ways in which the methodologies and predictions are shared. This leads to considerable difficulties for users trying to navigate the selection and application of VEPs. Here, to address these issues, we provide guidelines and recommendations for the release of novel VEPs.
PUBMED: 40234898 PMC: PMC11998465 DOI: 10.1186/s13059-025-03572-z
Benchmarking computational variant effect predictors by their ability to infer human traits.
Genome biology 2024;25;1;172
Computational variant effect predictors offer a scalable and increasingly reliable means of interpreting human genetic variation, but concerns of circularity and bias have limited previous methods for evaluating and comparing predictors. Population-level cohorts of genotyped and phenotyped participants that have not been used in predictor training can facilitate an unbiased benchmarking of available methods. Using a curated set of human gene-trait associations with a reported rare-variant burden association, we evaluate the correlations of 24 computational variant effect predictors with associated human traits in the UK Biobank and All of Us cohorts.
PUBMED: 38951922 PMC: PMC11218265 DOI: 10.1186/s13059-024-03314-7
Minimum information and guidelines for reporting a multiplexed assay of variant effect.
Genome biology 2024;25;1;100
Multiplexed assays of variant effect (MAVEs) have emerged as a powerful approach for interrogating thousands of genetic variants in a single experiment. The flexibility and widespread adoption of these techniques across diverse disciplines have led to a heterogeneous mix of data formats and descriptions, which complicates the downstream use of the resulting datasets. To address these issues and promote reproducibility and reuse of MAVE data, we define a set of minimum information standards for MAVE data and metadata and outline a controlled vocabulary aligned with established biomedical ontologies for describing these experimental designs.
PUBMED: 38641812 PMC: PMC11027375 DOI: 10.1186/s13059-024-03223-9
Characterizing glucokinase variant mechanisms using a multiplexed abundance assay.
Genome biology 2024;25;1;98
Amino acid substitutions can perturb protein activity in multiple ways. Understanding their mechanistic basis may pinpoint how residues contribute to protein function. Here, we characterize the mechanisms underlying variant effects in human glucokinase (GCK) variants, building on our previous comprehensive study on GCK variant activity.
PUBMED: 38627865 PMC: PMC11021015 DOI: 10.1186/s13059-024-03238-2
Benchmarking splice variant prediction algorithms using massively parallel splicing assays.
Genome biology 2023;24;1;294
Variants that disrupt mRNA splicing account for a sizable fraction of the pathogenic burden in many genetic disorders, but identifying splice-disruptive variants (SDVs) beyond the essential splice site dinucleotides remains difficult. Computational predictors are often discordant, compounding the challenge of variant interpretation. Because they are primarily validated using clinical variant sets heavily biased to known canonical splice site mutations, it remains unclear how well their performance generalizes.
PUBMED: 38129864 PMC: PMC10734170 DOI: 10.1186/s13059-023-03144-z
Cross-protein transfer learning substantially improves disease variant prediction.
Genome biology 2023;24;1;182
Genetic variation in the human genome is a major determinant of individual disease risk, but the vast majority of missense variants have unknown etiological effects. Here, we present a robust learning framework for leveraging saturation mutagenesis experiments to construct accurate computational predictors of proteome-wide missense variant pathogenicity.
PUBMED: 37550700 PMC: PMC10408151 DOI: 10.1186/s13059-023-03024-6
mutscan-a flexible R package for efficient end-to-end analysis of multiplexed assays of variant effect data.
Genome biology 2023;24;1;132
Multiplexed assays of variant effect (MAVE) experimentally measure the effect of large numbers of sequence variants by selective enrichment of sequences with desirable properties followed by quantification by sequencing. mutscan is an R package for flexible analysis of such experiments, covering the entire workflow from raw reads up to statistical analysis and visualization. The core components are implemented in C++ for efficiency. Various experimental designs are supported, including single or paired reads with optional unique molecular identifiers. To find variants with changed relative abundance, mutscan employs established statistical models provided in the edgeR and limma packages. mutscan is available from https://github.com/fmicompbio/mutscan .
PUBMED: 37264470 PMC: PMC10236832 DOI: 10.1186/s13059-023-02967-0
High-throughput deep learning variant effect prediction with Sequence UNET.
Genome biology 2023;24;1;110
Understanding coding mutations is important for many applications in biology and medicine but the vast mutation space makes comprehensive experimental characterisation impossible. Current predictors are often computationally intensive and difficult to scale, including recent deep learning models. We introduce Sequence UNET, a highly scalable deep learning architecture that classifies and predicts variant frequency from sequence alone using multi-scale representations from a fully convolutional compression/expansion architecture. It achieves comparable pathogenicity prediction to recent methods. We demonstrate scalability by analysing 8.3B variants in 904,134 proteins detected through large-scale proteomics. Sequence UNET runs on modest hardware with a simple Python package.
PUBMED: 37161576 PMC: PMC10169183 DOI: 10.1186/s13059-023-02948-3
A comprehensive map of human glucokinase variant activity.
Genome biology 2023;24;1;97
Glucokinase (GCK) regulates insulin secretion to maintain appropriate blood glucose levels. Sequence variants can alter GCK activity to cause hyperinsulinemic hypoglycemia or hyperglycemia associated with GCK-maturity-onset diabetes of the young (GCK-MODY), collectively affecting up to 10 million people worldwide. Patients with GCK-MODY are frequently misdiagnosed and treated unnecessarily. Genetic testing can prevent this but is hampered by the challenge of interpreting novel missense variants.
PUBMED: 37101203 PMC: PMC10131484 DOI: 10.1186/s13059-023-02935-8
satmut_utils: a simulation and variant calling package for multiplexed assays of variant effect.
Genome biology 2023;24;1;82
The impact of millions of individual genetic variants on molecular phenotypes in coding sequences remains unknown. Multiplexed assays of variant effect (MAVEs) are scalable methods to annotate relevant variants, but existing software lacks standardization, requires cumbersome configuration, and does not scale to large targets. We present satmut_utils as a flexible solution for simulation and variant quantification. We then benchmark MAVE software using simulated and real MAVE data. We finally determine mRNA abundance for thousands of cystathionine beta-synthase variants using two experimental methods. The satmut_utils package enables high-performance analysis of MAVEs and reveals the capability of variants to alter mRNA abundance.
PUBMED: 37081510 PMC: PMC10116734 DOI: 10.1186/s13059-023-02922-z
DIMPLE: deep insertion, deletion, and missense mutation libraries for exploring protein variation in evolution, disease, and biology.
Genome biology 2023;24;1;36
Insertions and deletions (indels) enable evolution and cause disease. Due to technical challenges, indels are left out of most mutational scans, limiting our understanding of them in disease, biology, and evolution. We develop a low cost and bias method, DIMPLE, for systematically generating deletions, insertions, and missense mutations in genes, which we test on a range of targets, including Kir2.1. We use DIMPLE to study how indels impact potassium channel structure, disease, and evolution. We find deletions are most disruptive overall, beta sheets are most sensitive to indels, and flexible loops are sensitive to deletions yet tolerate insertions.
PUBMED: 36829241 PMC: PMC9951526 DOI: 10.1186/s13059-023-02880-6
Leveraging massively parallel reporter assays for evolutionary questions.
Genome biology 2023;24;1;26
A long-standing goal of evolutionary biology is to decode how gene regulation contributes to organismal diversity. Doing so is challenging because it is hard to predict function from non-coding sequence and to perform molecular research with non-model taxa. Massively parallel reporter assays (MPRAs) enable the testing of thousands to millions of sequences for regulatory activity simultaneously. Here, we discuss the execution, advantages, and limitations of MPRAs, with a focus on evolutionary questions. We propose solutions for extending MPRAs to rare taxa and those with limited genomic resources, and we underscore MPRA's broad potential for driving genome-scale, functional studies across organisms.
PUBMED: 36788564 PMC: PMC9926830 DOI: 10.1186/s13059-023-02856-6
Saturation-scale functional evidence supports clinical variant interpretation in Lynch syndrome.
Genome biology 2022;23;1;266
Lynch syndrome (LS) is a cancer predisposition syndrome affecting more than 1 in every 300 individuals worldwide. Clinical genetic testing for LS can be life-saving but is complicated by the heavy burden of variants of uncertain significance (VUS), especially missense changes.
PUBMED: 36550560 PMC: PMC9773515 DOI: 10.1186/s13059-022-02839-z
MAVE-NN: learning genotype-phenotype maps from multiplex assays of variant effect.
Genome biology 2022;23;1;98
Multiplex assays of variant effect (MAVEs) are a family of methods that includes deep mutational scanning experiments on proteins and massively parallel reporter assays on gene regulatory sequences. Despite their increasing popularity, a general strategy for inferring quantitative models of genotype-phenotype maps from MAVE data is lacking. Here we introduce MAVE-NN, a neural-network-based Python package that implements a broadly applicable information-theoretic framework for learning genotype-phenotype maps-including biophysically interpretable models-from MAVE datasets. We demonstrate MAVE-NN in multiple biological contexts, and highlight the ability of our approach to deconvolve mutational effects from otherwise confounding experimental nonlinearities and noise.
PUBMED: 35428271 PMC: PMC9011994 DOI: 10.1186/s13059-022-02661-7
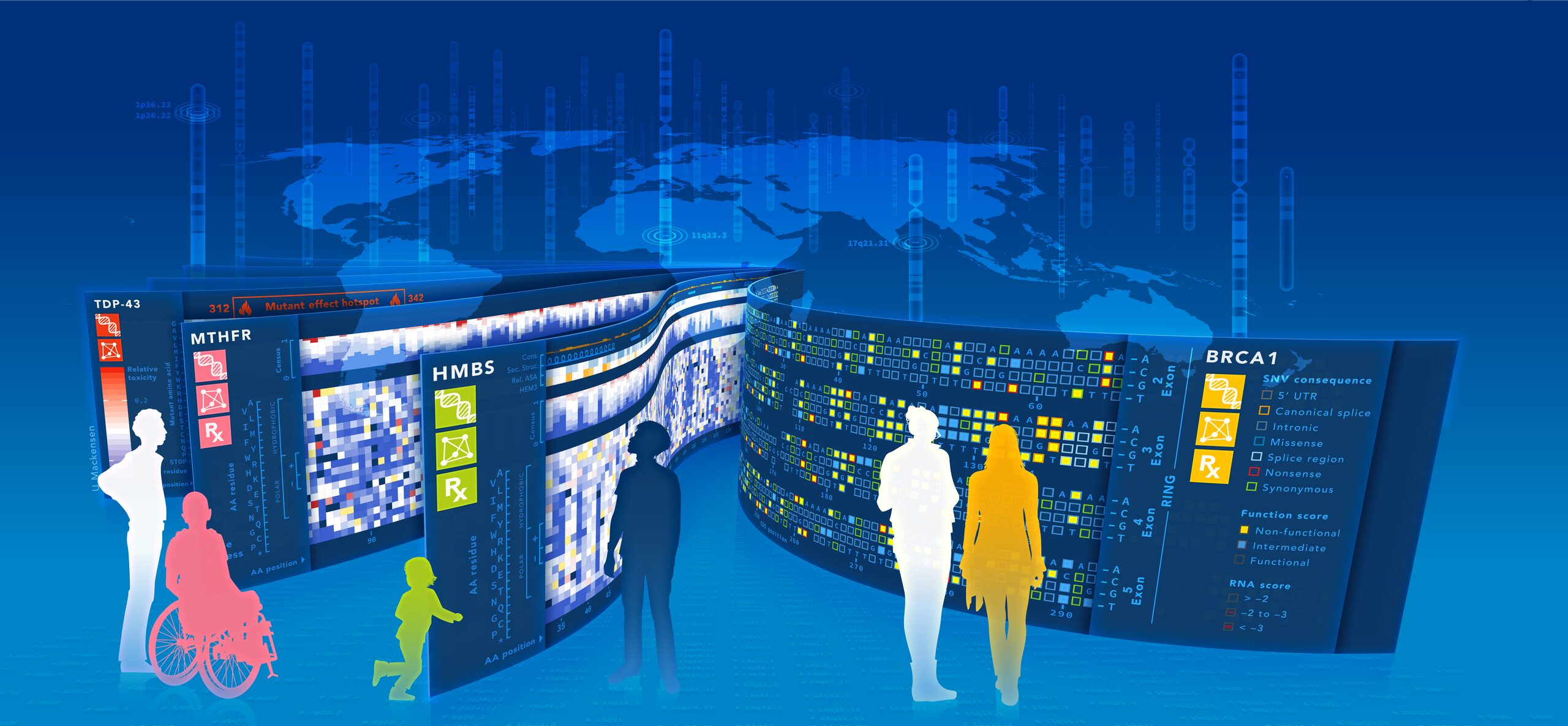